Table Of Content
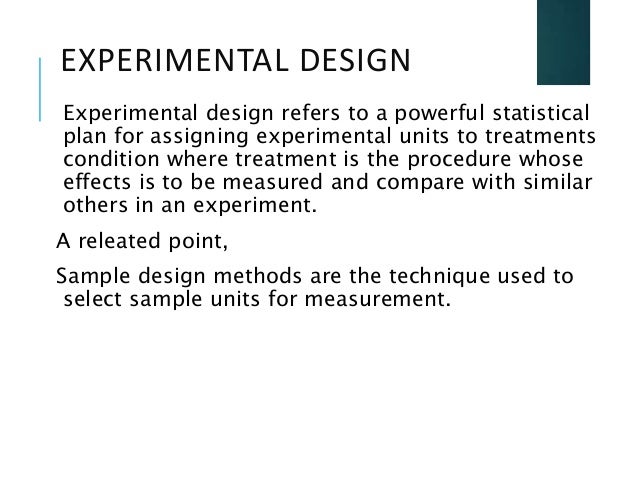
ANOVA is a statistical technique used to compare means across two or more groups in order to determine whether there are significant differences between the groups. There are several types of ANOVA, including one-way ANOVA, two-way ANOVA, and repeated measures ANOVA. Inferential statistics are used to make inferences or generalizations about a larger population based on the data collected in the study. Descriptive statistics are used to summarize and describe the data collected in the study. This includes measures such as mean, median, mode, range, and standard deviation. This involves systematically varying the order in which participants receive treatments or interventions in order to control for order effects.
Analysis of variance and significance testing
Of course, if you are participating in a study and you know that you are receiving a pill which contains no actual medication, then the power of suggestion is no longer a factor. Blinding in a randomized experiment preserves the power of suggestion. When a person involved in a research study is blinded, he does not know who is receiving the active treatment(s) and who is receiving the placebo treatment. A double-blind experiment is one in which both the subjects and the researchers involved with the subjects are blinded. A within-subjects design differs from a between-subjects design in that the same subjects perform at all levels of the independent variable.
What is the Scientific Method?
A single-blind experiment is when the subjects are unaware of which treatment they are receiving, but the investigator measuring the responses knows what treatments are going to which subject. In other words, the researcher knows which individual gets the placebo and which ones receive the experimental treatment. One major pitfall for this type of design is that the researcher may consciously or unconsciously influence the subject since they know who is receiving treatment and who isn’t. For many designed studies, the sample size is an integer multiple of the total number of treatments. This integer is the number of times each treatment being repeated and one complete repitition of all treatments (under similar experimental conditions) is called a complete replicate of the experiment.
4 Experimental units
Regression analysis is used to model the relationship between two or more variables in order to determine the strength and direction of the relationship. There are several types of regression analysis, including linear regression, logistic regression, and multiple regression. This design involves grouping participants within larger units, such as schools or households, and then randomly assigning these units to different treatment groups. A completely randomized design is the process of assigning subjects to control and treatment groups using probability, as seen in the flow diagram below.
Advantage of Within-Subjects Designs
Within-subjects designs are often called "repeated-measures" designs since repeated measurements are taken for each subject. Similarly, a within-subject variable can be called a repeated-measures factor. Counterbalancing (randomising or reversing the order of treatments among subjects) is often used in within-subjects designs to ensure that the order of treatment application doesn’t influence the results of the experiment.
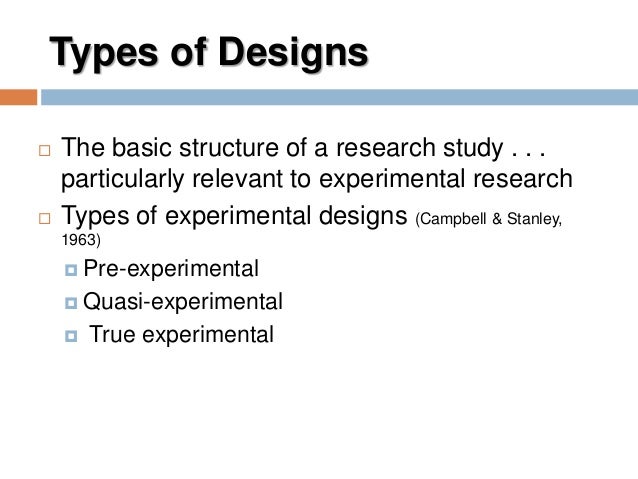
Data for statistical studies are obtained by conducting either experiments or surveys. Experimental design is the branch of statistics that deals with the design and analysis of experiments. The methods of experimental design are widely used in the fields of agriculture, medicine, biology, marketing research, and industrial production. A lurking variable is usually unobserved at the time of the study, which influences the association between the two variables of interest.
The treatments are given to two groups that can be matched up with each other in some ways. One example would be to measure the effectiveness of a muscle relaxer cream on the right arm and the left arm of individuals, and then for each individual you can match up their right arm measurement with their left arm. Another example of this would be before and after experiments, such as weight before and weight after a diet. Carefully assign subjects to different treatment groups, so that those given each treatment are similar in ways that are important to the experiment.
Doing this helps to account for the placebo effect, which is where a person’s mind makes their body respond to a treatment because they think they are taking the treatment when they are not really taking the treatment. Note, not every experiment needs a placebo, such when using animals or plants. As an example, if you are testing a new blood pressure medication you can’t give a person with high blood pressure a placebo or no treatment because of moral reasons. When a treatment is repeated under the same experimental conditions, any difference in the response from prior responses for the same treatment is due to random errors.
Regression and correlation analysis
Falsified data taints over 55 papers he authored and 10 Ph.D. dissertations that he supervised. Without proper controls and safeguards, unintended consequences can ruin our study and lead to wrong conclusions. One last consideration is the time period that you are collecting the data over. Except where otherwise noted, content on this site is licensed under a CC BY-NC 4.0 license. If your study system doesn’t match these criteria, there are other types of research you can use to answer your research question. Have a human editor polish your writing to ensure your arguments are judged on merit, not grammar errors.
Analyzing the data from this experiment reveals that the ratings in the charismatic-teacher condition were higher than those in the punitive-teacher condition. Using inferential statistics, it can be calculated that the probability of finding a difference as large or larger than the one obtained if the treatment had no effect is only \(0.018\). Therefore it seems likely that the treatment had an effect and it is not the case that all differences were chance differences. It is best that a process be in reasonable statistical control prior to conducting designed experiments.
An experiment might have just one independent variable or it might have several. This section describes basic experimental designs and their advantages and disadvantages. Experimental design provides a structured approach to designing and conducting experiments, ensuring that the results are reliable and valid.
However, because laboratory conditions are not always representative of real-world conditions, the results of these experiments may not be generalizable to the population at large. Textbook content produced by OpenStax is licensed under a Creative Commons Attribution License . The OpenStax name, OpenStax logo, OpenStax book covers, OpenStax CNX name, and OpenStax CNX logo are not subject to the Creative Commons license and may not be reproduced without the prior and express written consent of Rice University. Learning the basic theory of statistics will empower you to analyze statistical studies critically. Blind study is where the individual does not know which treatment they are getting or if they are getting the treatment or a placebo. An experiment is when the investigator changes a variable or imposes a treatment to determine its effect.
Okay, so using the example above, notice that one of the groups did not receive treatment. This group is called a control group and acts as a baseline to see how a new treatment differs from those who don’t receive treatment. Typically, the control group is given something called a placebo, a substance designed to resemble medicine but does not contain an active drug component. A placebo is a dummy treatment, and should not have a physical effect on a person.
Thinking About Experimental Design by Lance Zeng - Towards Data Science
Thinking About Experimental Design by Lance Zeng.
Posted: Tue, 28 Jul 2020 07:00:00 GMT [source]
The course covers best practices in experimental design and statistical analysis, ensuring scientific rigor and reproducibility. The explanatory variable is whether the subject received either no treatment or a high dose of vitamin C. The response variable is whether the subject had a seizure during the time of the study. The experimental units in this study are the subjects who recently had a seizure.
In a study of the effects of colors and prices on sales of cars, the factors being studied are color (qualitative variable) and price (quantitative variable). Specify how you can manipulate the factor and hold all other conditions fixed, to insure that these extraneous conditions aren't influencing the response you plan to measure. In your research design, it’s important to identify potential confounding variables and plan how you will reduce their impact. Use arrows to show the possible relationships between variables and include signs to show the expected direction of the relationships.
No comments:
Post a Comment